
Both the Fisher’s linear discriminant analysis and ANN can effectively predict climatic regions with multiple climatic variables. The best k-mean clustering suggested 9 clusters with more temperature clusters. The 4-means clusters include wet no freeze, dry no freeze, dry freeze and snow freeze. Results of PCA and factor analysis show that temperature and humidity are the first two principle components and common factors, accounting for 71.6% of the variance. Then, two supervised machine learning methods including Fisher’s discriminant analysis and Artificial Neural Networks (ANN) were adopted to predict the climatic regions based on climatic data. Three unsupervised machine learning including Principle Component Analysis (PCA), factor analysis and cluster analysis were conducted to identify the main component and common factors for climatic variables, and then to classify datasets into different groups. Around one third of the 800 weather stations record variation of freeze and precipitation classifications and a few of them show significant change of classifications over time based on the results of logistic regression analyses. It was found that both the temperature and humidity increased in most States. The effect and significance of climate change were firstly evaluated using time as the only predictor and t-test. The pivot view lets users find research projects that are completed, in progress, or planned using LTPP data.This study extracted 16 climatic data variables including annual temperature, freeze thaw, precipitation, and snowfall conditions from the Long-term Pavement Performance (LTPP) program database to evaluate the climatic regionalization for pavement infrastructure. The list view tab of this feature allows users to view and prioritize research reports on the basis of different attributes and selection criteria. This feature has two tabs: list view and pivot view. The LTPP Data Analysis Plan on LTPP InfoPave™ allows access to past research reports and projects.

The Expanded Data Analysis Plan, which is an up-to-date version of the Strategic Plan, shows ongoing, completed, and anticipated near-term LTPP data analysis projects, as well as closely related National Cooperative Highway Research Program ( NCHRP) projects.
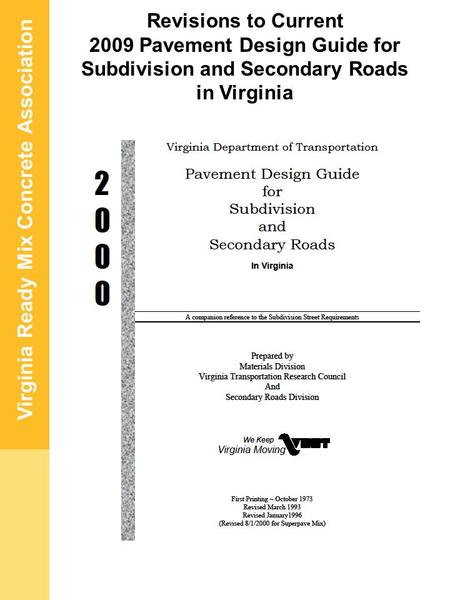
The plan sets forth strategic objectives supporting the goal "to develop knowledge, relationships, and models to facilitate improved pavement design and reliable performance predictions." Each strategic objective, in turn, is supported by several more focused "product objectives," which are color-coded to represent criticality and numbered to indicate sequencing. Since 1999, the national level analysis of the LTPP data has been guided by the “ Strategic Plan for Long-Term Pavement Performance Data Analysis.” The Strategic Plan was developed by the Transportation Research Board (TRB) Expert Task Group on LTPP Data Analysis, recommended by the TRB LTPP Committee and adopted by the Federal Highway Administration (FHWA) as the basis for selecting LTPP analysis projects and evaluating progress in the program's data analysis activities. All analysis findings provide valuable insight and direction to guide future LTPP data collection and analysis efforts.
#Ltpp rigid pavement design software software#
Some analysis findings have led to the development of products, such as the Rigid Pavement Design software and LTPPBind. The projects address a broad array of topics-from field validation of pavement design procedures, to studies of variability in traffic and materials data, to the investigation of the development of pavement roughness. The Long-Term Pavement Performance (LTPP) Program data analysis projects takes the raw data collected from the program's more than 2,500 pavement test sections and converts them into useable information.
